The rewards and risks of investing in GenAI for proposal development
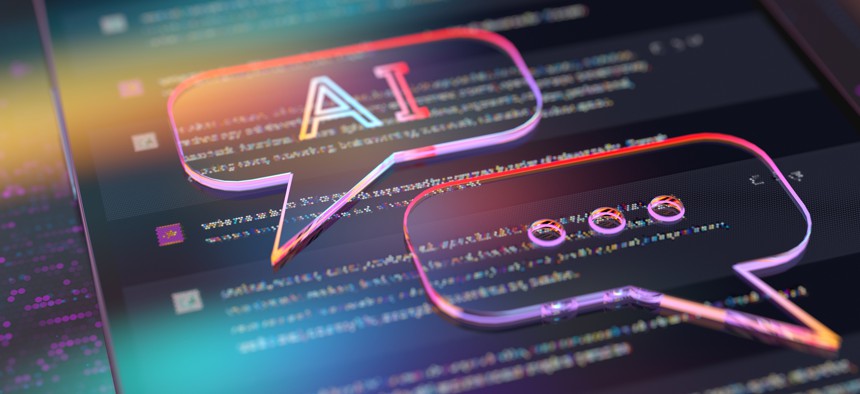
Gettyimages.com/Vertigo3d
Companies must weigh multiple factors when deciding how and what to invest in when picking a path forward with generative artificial intelligence for proposal development, writes Bruce Feldman of Lohfeld Consulting Group.
The rapid adoption of generative artificial intelligence for a wide range of business use cases creates a new challenge for chief financial officers:
How to assess, measure, and respond to the potential returns, costs, and risks from a whole new class of tools.
This article provides background and insight for CFOs to help address this problem.
- Explains how GenAI is used for proposal development.
- Describes how GenAI adoption can enhance revenue with higher submits volume from lowered proposal development costs and increased win rates from improved bid quality.
- Describes how to reduce the cost to acquire GenAI capability through a Make/Buy/Lease decision, platform type, and hosting (on-premise versus virtual private cloud) selection.
- Summarizes the key risks and mitigations associated with GenAI adoption.
GenAI is unique in that it can create previously non-existent content that is plausible and coherent.
While AI tools have been around for decades, GenAI exploded into public awareness in late 2022 as the emergent property of these tools — to create new, well-written content in response to a request and as part of an extended conversation — became apparent.
The rapid adoption of GenAI is evident not only from industry surveys but also in the startlingly rapid and widespread appearance of GenAI-created narratives, visual arts, music and software code outputs in social media and elsewhere.
GenAI use cases for businesses have multiplied so quickly that a major cautionary note in GenAI adoption is the need to maintain focus on your core use cases. A separate Lohfeld Consulting survey of GovCon companies found that approximately 75% of respondents have started on their GenAI adoption journey.
While it’s a foregone conclusion that GenAI offers the potential for significant gains in productivity and efficiency, it’s questionable whether the return from investment can be calculated in entirely the same way as software or other elements of corporate infrastructure.
Acquiring and sustaining a GenAI capability injects new and different costs into your workplace IT operations.
Using GenAI for Proposal Development
GenAI is most impactful in reducing the time and effort it takes to make your proposal compliant and responsive because GenAI tools allow users to generate high-quality draft proposal content in response to user requests in seconds.
Such requests are called prompts and are written in plain English (or any other language in which the GenAI is trained). With a GenAI chatbot, prompts allow the user to have a conversation with the AI, alternating requests and responses. In the prompt, the user can direct the content, role, structure, style, tone, length and format of the GenAI response.
GenAI platforms write fluently with high-quality grammar and syntax. GenAI platforms can also include AI-assisted tools for specific tasks. It is notable, however, that the inherent creativity of GenAI platforms makes them unreliable for use cases that require 100% accuracy or repeatable outcomes[1]. The user (e.g., the task owner) must treat GenAI responses as drafts to be fact-checked and then reviewed and revised into final, polished prose.
Improving Financial Performance with GenAI
Under the heading of “You can’t spend your way to prosperity,” the enterprise focus for GenAI adoption needs to be revenue improvement, with secondary consideration of cost savings that go to the bottom line. Revenue improvement from using GenAI for business development comes from two sources: (1) increased number of proposal submissions and (2) increased win rate.
Lohfeld Consulting’s experience with GovCon customers leads us to estimate that a proposal development workforce with moderate proficiency in a GenAI platform can realize productivity gains of 30% or better. These efficiencies are primarily made in reduced labor hours.
In principle, a 30% reduction in average proposal hours would provide the resources for a 40% increase in the number of proposals submitted (assuming those resources remain in place). There is thus an immediate potential for a 40% increase in awards, with consequent revenue impact (assuming win rates are sustained).
Some vendors claim efficiencies of about 80% or better; however, Lohfeld Consulting suspects such elevated levels of performance will not be immediately available, as they require a highly proficient workforce and significant investment in organizational change management.
Our experience with GenAI suggests that proposal quality can improve as well, sustaining or improving win rates for revenue enhancement. Sustaining and improving proposal quality is critical for two reasons: (1) revenue and profit growth require winning, not merely bidding, and (2) federal, state, and local governments have shown concern and may take steps to foreclose the potential for being inundated with mediocre GenAI-created submissions.
Of the three benchmarks for proposal development — compliant, responsive, and compelling — GenAI is most impactful in reducing the time and effort to be compliant and responsive. GenAI can contribute to reaching a compelling response, but the human factor in performing Strength-Based Winning is most important here.
Lohfeld Consulting is already teaching a class and training its industry clients on how to use GenAI to enable their professional staff to create the high-quality, feature-rich narratives needed to outscore competitors, improving win rates.
Reduced Opportunity Cost
An additional savings from GenAI comes from reducing the diversion of subject matter expert talent from programs that generate revenue from labor — an opportunity cost. Companies strain their people (and customer relationships) when they divert their top performers to proposal work.
Reducing the time that your workforce, especially your top technical talent, spends on proposal work reduces strain on the enterprise. The most stressful case is when a company decides to build its own GenAI “foundational model,” choosing to divert resident AI, data science, and IT staff away from revenue-generating projects and go-to-market to in-house tool development.
It is a truism that keeping your top people engaged with your customers also improves project team morale and customer relations.
Initial Investment in a GenAI Platform – Make/Buy/Lease
The impact of acquiring a GenAI platform will be felt primarily on cash flow, as determined by the allocation of cost in capital expenditure and amortization. This decision will include your choice for hosting the GenAI platform — whether on-premise (in your in-house IT infrastructure) or with the data and applications you already host in your virtual private cloud.
Make. The “Make” paradigm allows the user to take responsibility for developing key components of the GenAI architecture. This responsibility can vary from minimal, such as building a user interface to a public model (such as OpenAI’s ChatGPT-4 or Google Gemini), to end-to-end development of a GenAI platform, including what is typically a very expensive and complex Large Language Model.
McKinsey has defined three archetypes that illustrate classes of in-house models, with an associated cost range for initial development and installation. This approach gives valuable insight into the “Make” tradespace.
It is our observation that the AI practice within a company often wants to take on responsibility for developing the “Make” components. The cautionary note for proceeding down this path is the diversion of resources away from developing capabilities needed for go-to-market and the learning curve expense when compared to outsourcing the capability to a vendor that has already developed a suitable capability.
Buy. The “Buy” option of purchasing a model offers considerable time, expenditure, and opportunity cost savings over developing a model in-house since the vendor (presumably) has the advantage of economies of scale and external investment to defray development costs. The cost for initial investment is much lower. The tradeoff is that the vendor’s standard product must be customized to your needs and environment.
Lease. The “Lease” option implies acquisition of the capability through Software as a Service (SaaS). This acquisition model outsources the development and operation of the GenAI model, so you have reduced initial investment and annual sustainment costs.
The standard product is deployed into your environment, and you must allow for vendor sustainment activity inside your cybersecurity regime. Leasing can provide service per seat, by token (usage), or other (e.g., fixed). Lohfeld Consulting’s experience is that the “Lease” alternative is much less expensive than “Make” or “Buy,” and the performance is more than acceptable.
Annual Sustainment Costs for Your GenAI Platform – Model Deployment
There is a range of expected annual run costs for each model archetype. These costs include the operating cost of the model (to generate outputs from inputs, called “model inference”) as well as model and interface maintenance. Hidden costs such as maintaining cognizance of GenAI platform evolution, managing technical debt, confirming compliance, and setting up and managing a Key Performance Indicator (KPI) program can also be expected.
Model Hosting – On-Premise versus in the Cloud. As of mid-2024, it appears that GovCon is converging on two alternative GenAI hosting models for their business development applications: (1) on the company resident IT infrastructure and (2) in a company’s virtual private cloud (VPC).
The March 2024 article from Andreesen Horowitz a16z[2] cites a survey of 70 enterprise decision-makers showing that 28% are hosting GenAI on their internal network and 72% access GenAI from a model provider endpoint accessed through a VPC. Both approaches allow you to take advantage of in-place cybersecurity measures.
Installation on a corporate network is expected to be more expensive, as it will require greater customization to integrate the GenAI software into your unique IT environment compared to hosting on your company’s virtual private cloud (e.g., Microsoft Azure).
Making Corporate Data Available to the GenAI Platform – Fine-Tuning vs. Retrieval Augmented Generation (RAG). The same survey cited in the Andreesen Horowitz a16z March 2024 article also shows how enterprise decision-makers are making their GenAI models knowledgeable of their company’s proprietary data with protection from corruption and leakage.
The article notes that the trend has shifted in just the last year from building GenAI models trained on company data in-house (à la BloombergGPT) to predominantly either fine-tuning models (72%) or using retrieval augmented generation (22%).
This choice — whether to use fine-tuning vs. RAG — has a major impact on sustainment cost. Fine-tuning delivers superior performance but involves recurring additional training of the GenAI’s Large Language Model, a complex and time-consuming activity that is performed by AI specialists.
Implementation and Risk Management for GenAI
GenAI does have significant risks and limitations on its performance, which can be mitigated at least partially with appropriate procedures and proper workforce preparation/training.
The main risks for the application of GenAI to business development are (1) the need to invest in the culture change and process updates to make the most effective use of GenAI, (2) the tendency to make assertions that are not true, called “hallucinations,” and (3) the risk of compromise of corporate data.
Hidden Cost of Organizational Change Management
A McKinsey report estimates that the investment in Organizational Change Management for GenAI might cost as much as three times the investment in the model itself.
This estimate seems high to the author, especially given the investment cost at the higher end of the model archetypes, but there is an essential truth here: a significant (and often overlooked) investment in OCM is required to help people who may have spent decades writing proposals to adapt to a new type of tool. OCM includes organizational familiarization, training, adaptation to and of your best practices, and building enterprise fluency and understanding of AI.
OCM also needs to address the impact on your company’s IT organization. New roles may emerge and need to be filled, and there may be existing roles that can be eliminated. There may also be tension with your company’s in-house AI practice when the choice is made to outsource the capability.
In a recent interview, Sam Altman, President of OpenAI, asserted that the next-generation workforce will have grown up with GenAI and will expect it as part of their office toolkit. If this happens, then delaying implementation will create a disincentive for hiring—an indirect source of cost.
Potential For Incorrect Assertions – Hallucinations
GenAI can produce text with incorrect or even entirely made-up assertions, creating the potential for corporate exposure. This phenomenon is called “hallucination” and is currently benchmarked at 2% - 4%, with rates as high as 27% having been observed, depending on the platform.
GenAI is a subject matter expert in domains in which it has been initially trained, but it is important to understand that GenAI is not using rules of logic or reasoning to generate its outputs. Rather, it uses the statistical relationships between the words in its training data to recommend future words; GenAI is a “word prediction engine.”
It is easy for users to become complacent in reading the high-quality writing that is available from a GenAI platform, but it is essential that users check all factual assertions and edit GenAI responses for nuance and intent, as a factually incorrect statement in a legally binding document can have catastrophic consequences.
Potential for Compromise of Corporate Data
GenAI must be able to use corporate proprietary data, intellectual property, and potentially personally identifiable information (PII) to create high-quality proposals. It is imperative that safeguards be in place to prevent this data from corruption and leakage.
Hosting GenAI on-premises or in your VPC provides the same cybersecurity resilience that you have for your data.
For those GenAI platforms that securely access a commercial GenAI endpoint outside your control (e.g., Microsoft Azure OpenAI Service), you have contractual assurance that your data is protected from corruption and leakage. Several GenAI platforms are FedRamp certified and approved by the Department of Defense to host Controlled Unclassified Information at Impact Level 4.
We strongly urge all companies to (1) implement policies that prohibit the use of public platforms whose vendors record their GenAI inputs and outputs and (2) educate their workforce accordingly. These vendors record input/response pairs for training future models. Inputting proprietary and other sensitive information into their public models may lead to incorporating and disclosing that information in future generations of the platform.
Conclusions
GenAI offers the potential to increase revenue through increased proposal workforce productivity and higher-scoring proposals. The adoption of GenAI, however, requires consideration of the costs, risks, and mitigations.
As described previously, the major drivers of cost and risk are (1) your Make/Buy/Lease decision, (2) your GenAI hosting decision, and (3) hidden costs for organizational change management and data management. You also need to keep in mind that GenAI is evolving at a frenetic pace.
Three general trends for the business application of GenAI are emerging:
- Fewer companies are building custom GenAI platforms for their own use. This is because building a GenAI platform is expensive, complicated, and time-consuming.
- More companies are giving GenAI platforms access to their data through fine-tuning and/or RAG rather than diverting in-house resources to build a model around corporate data.
- For GovCon domain-aware GenAI platforms, most platform developers deploy a SaaS approach, but there are vendors who will build and customize a model for you.
Bruce Feldman is a vice president leading Lohfeld Consulting’s AI initiative and brings 30+ years of business development, capture management, and proposal development expertise specializing in space and national intelligence programs for the Air Force), Space Force, intelligence community, Office of the Secretary of Defense, Department of Defense agencies, and combatant commands. He is a retired Lt. Col., USAFR and has a MSEE from the Massachusetts Institute of Technology, a BSEE from the Air Force Institute of Technology, and a BA in Chemistry from Yale University.