How technology can play a critical role in reducing suicides among active-duty personnel and vets
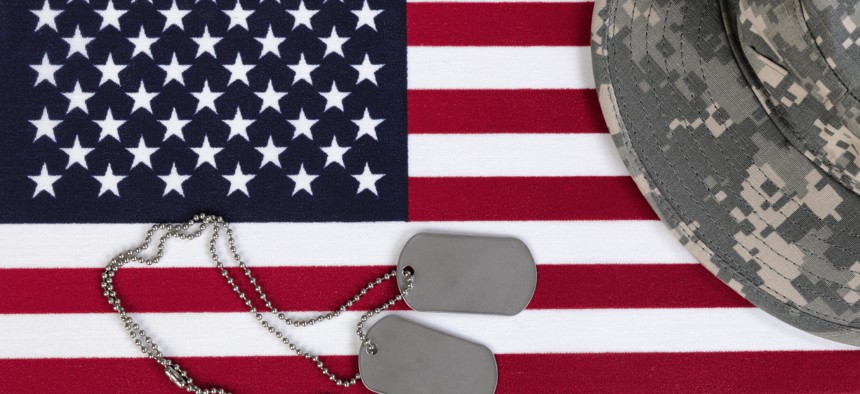
Gettyimages.com/ tab1962
Accenture executive Kelly Faddis and retired Air Force leader Tony Reardon explain the power of AI and machine learning to identify and support military personnel at risk of suicide.
Across the U.S. Air Force and, indeed, the entire U.S. military, suicides remain too pervasive. The same is true for the veterans population writ large, with an average of 17 veterans dying by suicide each day– resulting in a suicide rate, e.g. suicides per 100,000 individuals, that is nearly 72 percent higher than the suicide rate for non-veteran U.S. adults.
But new technologies are being explored to drive new thinking, actions, and policies that could make a difference.
One promising initiative is using artificial intelligence (AI) and machine learning (ML) to help mental health professionals identify military personnel who are at “significant risk” of suicidal behaviors.
Programs using predictive modeling to identify patients at risk of suicide are critically important, as providing care and treatment as early as possible significantly reduces risk of suicide. An analysis of one predictive effort initiated by the Veterans Administration in 2017 found the program reduced suicide attempts, mental health admissions, and emergency department visits among veterans.
Supporting Air Force Concepts Development & Management (CDM), we analyzed a set of civilian medical data in which names and other personal information were removed (this dataset contains only data compliant with the HIPAA de-identification standard).
This civilian data was massive and multi-layered, including information on chronic conditions, physical and mental health diagnoses, dates of treatment, location, and insurance status for 53 million patients globally, across 27 billion patient records covering 11 years.
A subset of the civilian data focused on U.S. adults diagnosed with depression, suicidal ideation, and other mental health conditions. These data were used to test and evaluate different AI/ML modeling techniques. The goal was to determine whether a machine learning model can accurately forecast suicidal behavior events.
Based on the lessons learned from the civilian study, Air Force CDM plans to extend the project to explore how the same technologies can provide insights into service members’ mental health via the Suicide Analytic Variable Evaluation System, or SAVES, project.
Although suicidal behaviors are complex and have no single cause, an analysis of the data can reveal trends, patterns, and location hot spots as to who is most at risk by their working conditions, gender, race, age, episodes or relapse of depression or drug addiction.
Consider, for example, a service member with a spotty credit record who, after losing a member of their unit while serving overseas, returns home and learns of a sudden death in the family. This sequence of events, when fed into the model, could trigger an alert to medical professionals that this individual is at risk of experiencing a suicidal behavior event.
With this information a medical professional assigned to a squadron could take actions such as pairing a service member with a therapist, recommending more time between missions to commanding officers, or suggesting a trip home to spend time with family.
While the use of AI/ML technologies shows great promise for suicide prevention, we must be vigilant in safeguarding data, both to ensure the reliability and accuracy of AI/ML models and to provide transparency in the use of data and in acquiring informed consent.
Our research is currently focused on developing AI/ML capabilities that are viable for predicting suicidal behaviors; success of this research must drive ethical and policy discussions regarding the implementation and management of these technologies prior to deployment.
The use of AI/ML to identify potential suicidal behaviors is showing promise based on early tests and analysis. Final decisions, however, on how it will be used and even if it should be used are still in the future.
Ensuring that patients and practitioners trust the technology and safeguards exist to prevent unintended consequences is critically important. That is why a parallel effort must take place to ensure the ethical use of this technology, as well as to determine who should have access to results, how individuals can opt out, and how to link the results with appropriate intervention.
These questions will be answered by medical professionals, ethicists, Air Force senior leaders, and AI/ML experts to ensure the technology is used consistently and without causing unintended consequences.
None of these questions are easy to address.
The technology, however, is powerful and there is reason to believe – based on science and rigorous testing – that it can help prevent suicides. If AI/ML is ultimately used, it is important to understand that this technology will only be one tool among others to assist trained medical professionals in diagnosing and treating those in need.
There is no single solution to prevent suicide. A comprehensive approach-- recognizing that mental health is health --is needed that provides multiple resources to at-risk individuals at the earliest opportunity. AI/ML can play a key role in bringing timely intervention options to people who need them most.
***
Where to find resources and support: Suicide and Crisis Lifeline (call 988) DOD Safe Helpline, 877-995-5247; National Suicide Prevention Helpline, 800-273-8255; and Veterans Crisis Line, 800-273-8255.
Anthony P. "Tony" Reardon, former senior executive service, administrative assistant to the Secretary of the Air Force, Headquarters U.S. Air Force (Ret.)
Kelly N. Faddis, Ph.D., Managing Director, Accenture Federal Services
*This article is published by the authors and not on behalf of the Department of the Air Force